Evolution of AI and its potential: Q&A with Google
Summary of a recent AI-focused panel discussion with senior leaders from Google Asia, moderated by Portfolio Manager, Richard Clode.
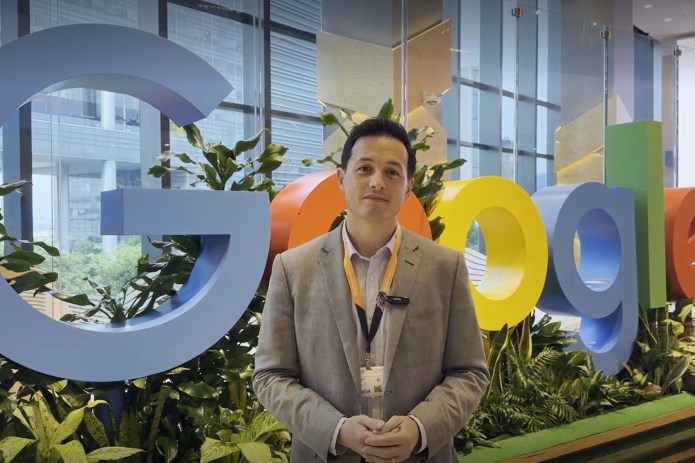
8 minute read
Key takeaways:
- Large language transformer models are unleashing generative AI, driving enormous customer interest.
- Hyperscalers are benefiting from the need for cloud infrastructure, the delivery mechanism for generative AI.
- Responsible disruption is becoming a focus, which is very positive for the tech sector’s long-term prospects.
Q: AI has been around for decades, why are we seeing a resurgence currently?
Many companies, including Google have been using AI for years to improve their products and services – be it more accurate Search results or suggesting better routes in Maps. Another common use of AI has been predictive text, for example in Gmail. Google pioneered the concept of transformer models as a technology, and open-sourced it. The GPT in ChatGPT is the acronym for generative pre-transformer.
Now, the fundamentals are in place to create the opportunities for AI. Processing speed is so much better. A tremendous amount of performance is required to train large language models with hundreds of billions of parameters. Second, the massive explosion in data is the fuel allowing these models to be able to produce even better results and outcomes.
At the same time, these large language models (LLMs) are being rapidly developed, they are getting smarter, and learning is accelerating. The possibilities are vast in terms of where generative AI (gen AI) can be applied. LLMs are powering generative AI. If you ask Google Search today, what is 2+2, it will return the answer as 4 not because it has intelligence, but because it’s appeared somewhere in a document that Google’s LLMs have been trained on.
It’s the predictive capabilities that are making AI so very interesting. Companies and organisations typically ask three key questions: How can we save costs with AI? How can my employees be more productive? How can the customer experience be improved? A digital support assistant is a real use case for generative AI, and as it becomes more embedded into processes, it gets more useful.
Also key is that the monetising of data that has begun. Application Programming Interfaces (APIs) that enable two software components (like an app and mobile phone) to communicate with each other were often free. Recently we’ve seen popular social platforms like Twitter and Reddit charging developers to access the data for their apps. Companies are looking to monetise their data and crucially, it prevents everyone from scraping the internet to create LLMs.
Q: Which areas have the most potential for AI applications?
In terms of consumers, the focus is on the tools and services that can make their lives better. There are a huge range of consumer interactions that are going to get a lot easier and become more immersive because of AI. Meanwhile, enterprise customers are using AI to supercharge business growth.
The third leg to this is responsibility, what are the opportunities to improve society as a whole? We need to protect people from the potential dangers of AI via safeguards and regulation. How can we make people’s lives better, how can AI do that much more efficiently? Some areas where AI is already assisting with are diagnosing disease at scale, assisting with flood forecasting, the US is seeing a record year for new drugs, with AI enabling that productivity.
Q: Where is generative AI being used today?
Every product from Google has AI embedded in it. Google is seeing real interest from consumers to interact with this technology and use it in interesting ways. There are multiple areas that are exciting and have huge potential with the help of AI. The fundamental human desire to search for information that is relevant and is semantic in nature, is very powerful. We can have an AI-assisted journey through an HR helpdesk, a bank helpdesk, a travel helpdesk, or any other customer-facing need. AI helps unlock data finding. Generative AI using large language models has the ability to naturally query any data source and it can be trained it in a particular way to suit.
Sundar Pichai, Google and Alphabet CEO said that their approach to AI has to be bold, but also responsible. How AI is developed and deployed can be for the betterment of society, be it the transformation of healthcare delivery, slowing down climate change and other ways that could ultimately make our lives better. Google’s Green Light project makes use of gen AI and driving trends to help curb auto emissions, by creating the most efficient traffic patterns and routing.
In terms of improving employee productivity, on average a software developer spends a minimum of 30 minutes a day looking for solutions. AI technology can help by speeding the process up, offering a solution within seconds and writing the code to deploy the solution. This use case for AI is already widespread and it broadens out to every industry, be it financial services, telcos, retail, healthcare, ecommerce, governments, etc.
Q: Why is the cloud a key delivery mechanism for AI? What is driving hyperscalers’ need to have specialised microchips that entail significant R&D costs?
Generative AI is not just one technology, it’s a stack of technologies, with the most fundamental being infrastructure. Andrew Ng of Stanford University said, “Artificial intelligence is the new electricity.” If we extend that phrase, data is the fuel that pumps the grid, and the grid is actually the cloud. Cloud data and AI are very closely interconnected; cloud hyperscalers are becoming core and centre of everything AI. Cloud is the infrastructure at the bottom, needed to manage, process and store large data sets. Uber Eats has powered its entire conversational AI interface using Google APIs and infrastructure. And importantly, big data is the catalyst for the cloud, because to truly rearchitect and be able to leverage gen AI, and make data accessible, you need cloud infrastructure.
There are three reasons justifying the need for bespoke chips: price-performance, sustainability, and the sheer complexity of LLMs to meet customers’ complex and custom needs. Google has partnered with NVIDIA on the GPU (graphics processing) side, but has also historically innovated on TPUs, or tensor processing units. Tensor Processing Units (TPUs) are Google’s custom-developed application-specific integrated circuits (ASICs) used to accelerate machine learning workloads.
Today’s general-purpose chips only perform standard multiplication, but complex large data sets (zettabytes of information) requires matrix multiplication, which standard CPUs (that run the operating system and apps) cannot do. That’s why the whole notion of accelerators started and are designed by the likes of nVIDIA and Marvell Technology. The largest large language model today for Google has about 540 billion parameters. The next one will have around a trillion parameters.
In terms of cost and sustainability factors, the more efficient you become, the cost to serve the query becomes lower. The training and inferencing costs for large language models are also extremely carbon intensive, hence the desire and need for hyperscalers to design their own silicon.
Q: What are the main concerns relating to AI given how powerful it can be?
Responsible disruption is incredibly important because it impacts people’s lives, jobs, governments and economies. Generally, tech companies are adopting a much more thoughtful approach around this compared to the past, which is very positive for the sector.
Security in terms of data privacy and cyber criminality are inherent with any new developing technology. What AI also brings is an explainability challenge. When a chatbot/conversational AI is asked a question and spurts out an answer, how does it explain how it arrived at that answer? This is an issue particularly impacting organisations where customers ultimately rely on trust, such as governments and banks.
Explainability is a tough problem in a large language model. When two humans interact it’s not that easy to predict how the other is going to respond because it depends on context, where and when they meet, etc. It’s the same situation when it comes to generative AI and large language models. Linked with this is ‘hallucinating’ when generative AI comes up with something completely invented.
Google’s Bard has incorporated a checking feature, which leverages on its strength in Search to check the facts, which aims to help make AI a more reassuring, positive experience. Identifying and taking down misinformation are areas that are a key focus.
In terms of proprietary data and scraping concerns, Google’s view is that access to large language models will be democratised, be it from Google, OpenAI, Meta etc. Large language models are typically trained on generic data that’s freely available. Enterprises gain real value when they combine their proprietary data with the power of a large language model. This is when they start to benefit, by integrating customer data within proprietary databases with front-end platforms, and back-end systems.
Note: Google senior leaders – Mitesh Agarwal, Chief Technology Officer, Google Cloud APAC and Simon Kahn, Chief Marketing Officer, Google APAC.
AI inference: the first phase of machine learning is the training phase where intelligence is developed by recording, storing, and labeling information. In the second phase, the inference engine applies logical rules to the knowledge base to evaluate and analyse new information, which can be used to augment human decision making.
Compute: relates to processing power, memory, networking, storage, and other resources required for the computational success of any programme.
CPU: the central processing unit is the control center that runs the machine’s operating system and apps by interpreting, processing and executing instructions from hardware and software programmes.
Generative AI: refers to deep-learning models that train on large volumes of raw data to generate ‘new content’ including text, images, audio and video.
GPU: a graphics processing unit performs complex mathematical and geometric calculations that are necessary for graphics rendering.
Hyperscalers: companies that provide infrastructure for cloud, networking, and internet services at scale. Examples include Google Cloud, Microsoft Azure, Meta Platforms, Alibaba Cloud, and Amazon Web Services (AWS).
LLM (large language model): a specialised type of artificial intelligence that has been trained on vast amounts of text to understand existing content and generate original content.
Open source software: code that is designed to be publicly accessible, in terms of viewing, modifying and distributing.
TPU: the primary task for Tensor Processing Units is matrix processing, which is a combination of multiply and accumulate operations. TPUs contain thousands of multiply-accumulators that are directly connected to each other to form a large physical matrix.
Transformer model: a neural network that learns context and thus meaning by tracking relationships in sequential data.
IMPORTANT INFORMATION
Technology industries can be significantly affected by obsolescence of existing technology, short product cycles, falling prices and profits, competition from new market entrants, and general economic conditions. A concentrated investment in a single industry could be more volatile than the performance of less concentrated investments and the market.
These are the views of the author at the time of publication and may differ from the views of other individuals/teams at Janus Henderson Investors. References made to individual securities do not constitute a recommendation to buy, sell or hold any security, investment strategy or market sector, and should not be assumed to be profitable. Janus Henderson Investors, its affiliated advisor, or its employees, may have a position in the securities mentioned.
Past performance does not predict future returns. The value of an investment and the income from it can fall as well as rise and you may not get back the amount originally invested.
The information in this article does not qualify as an investment recommendation.
Marketing Communication.
Important information
Please read the following important information regarding funds related to this article.
- Shares/Units can lose value rapidly, and typically involve higher risks than bonds or money market instruments. The value of your investment may fall as a result.
- Shares of small and mid-size companies can be more volatile than shares of larger companies, and at times it may be difficult to value or to sell shares at desired times and prices, increasing the risk of losses.
- If a Fund has a high exposure to a particular country or geographical region it carries a higher level of risk than a Fund which is more broadly diversified.
- The Fund is focused towards particular industries or investment themes and may be heavily impacted by factors such as changes in government regulation, increased price competition, technological advancements and other adverse events.
- The Fund follows a sustainable investment approach, which may cause it to be overweight and/or underweight in certain sectors and thus perform differently than funds that have a similar objective but which do not integrate sustainable investment criteria when selecting securities.
- The Fund may use derivatives with the aim of reducing risk or managing the portfolio more efficiently. However this introduces other risks, in particular, that a derivative counterparty may not meet its contractual obligations.
- If the Fund holds assets in currencies other than the base currency of the Fund, or you invest in a share/unit class of a different currency to the Fund (unless hedged, i.e. mitigated by taking an offsetting position in a related security), the value of your investment may be impacted by changes in exchange rates.
- When the Fund, or a share/unit class, seeks to mitigate exchange rate movements of a currency relative to the base currency (hedge), the hedging strategy itself may positively or negatively impact the value of the Fund due to differences in short-term interest rates between the currencies.
- Securities within the Fund could become hard to value or to sell at a desired time and price, especially in extreme market conditions when asset prices may be falling, increasing the risk of investment losses.
- The Fund could lose money if a counterparty with which the Fund trades becomes unwilling or unable to meet its obligations, or as a result of failure or delay in operational processes or the failure of a third party provider.
Specific risks
- Shares/Units can lose value rapidly, and typically involve higher risks than bonds or money market instruments. The value of your investment may fall as a result.
- If a Fund has a high exposure to a particular country or geographical region it carries a higher level of risk than a Fund which is more broadly diversified.
- The Fund is focused towards particular industries or investment themes and may be heavily impacted by factors such as changes in government regulation, increased price competition, technological advancements and other adverse events.
- This Fund may have a particularly concentrated portfolio relative to its investment universe or other funds in its sector. An adverse event impacting even a small number of holdings could create significant volatility or losses for the Fund.
- The Fund may use derivatives with the aim of reducing risk or managing the portfolio more efficiently. However this introduces other risks, in particular, that a derivative counterparty may not meet its contractual obligations.
- If the Fund holds assets in currencies other than the base currency of the Fund, or you invest in a share/unit class of a different currency to the Fund (unless hedged, i.e. mitigated by taking an offsetting position in a related security), the value of your investment may be impacted by changes in exchange rates.
- When the Fund, or a share/unit class, seeks to mitigate exchange rate movements of a currency relative to the base currency (hedge), the hedging strategy itself may positively or negatively impact the value of the Fund due to differences in short-term interest rates between the currencies.
- Securities within the Fund could become hard to value or to sell at a desired time and price, especially in extreme market conditions when asset prices may be falling, increasing the risk of investment losses.
- The Fund could lose money if a counterparty with which the Fund trades becomes unwilling or unable to meet its obligations, or as a result of failure or delay in operational processes or the failure of a third party provider.